
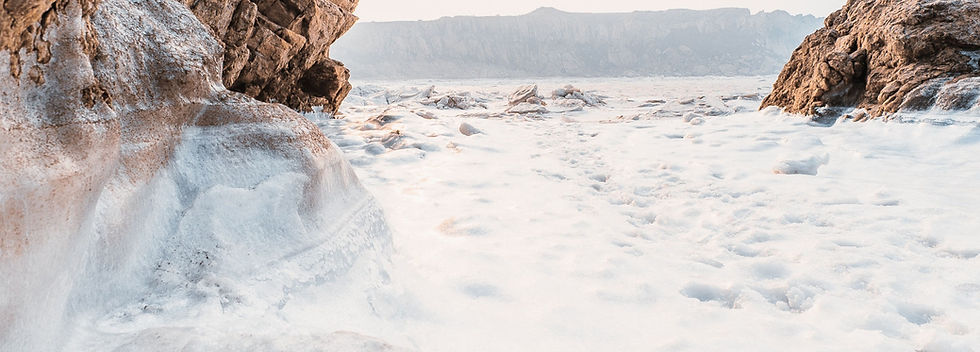
PhD Project
PhD Project: Inference of large chemical networks & applications to origin of life and astrochemistry
Context. Many chemists have been confronted, since the groundbreaking Miller–Urey experiment in 1952 or even before, with the difficulty of dealing with large chemical reaction networks comprising hundreds of molecule types or more. Such networks arise in particular in a prebiotic context. The Miller–Urey experiment demonstrated the synthesis of a large diversity of organic molecules from inorganic components, and set out a vast research program aiming at understanding physico-chemical conditions and processes having led to the emergence of life on the early Earth, through a sequence of mostly unknown evolution steps. With the multiplication of observations of exoplanets since 2004, the interest has broadened to the discussion of possible biosignatures attesting to the presence of life elsewhere in the Universe.
Depending on research groups, emphasis has been put either on RNA-first or metabolism-first scenarios. In both cases, the key element to be demonstrated is an evolution mechanism leading to more complex molecules or molecule networks, which could possibly be extrapolated to extant biological systems. In this respect, autocatalytic processes, characterized by linear instabilities of the underlying equations, are expected to play a prominent role.
​
Challenges. Detection of compounds proceeds through complex GC-MS (gas chromatography/mass spectroscopy) or LC/MS (liquid chromatography/mass spectroscopy) techniques.
The mass spectrum of a compound is in the form of a series of peaks. Databases provide only a tiny fraction of these signatures. For samples with a large diversity, only raw formulas are readily accessible; thus, it is impossible to write down a closed list of chemical compounds. Experiments clearly show several time phases, in which new compounds may appear, and then disappear, which are very difficult to interpret.
From the mathematical side, huge progress has been made very recently towards a general characterization of autocatalysis, and beyond that, a semi-quantitative description in terms of hierarchical models of the time behavior of generic chemical reaction networks under a scale-separation hypothesis.
​
Project. The main goal of the thesis is to fit mass spectroscopy data, derived by chemists interested in the origin of life, with the family of hierarchical models. An adequate statistical method will be built to infer parameters of the hierarchical models, which can be interpreted as proxy kinetic rates. Importantly, a Bayesian prior distribution on the kinetic parameters of potentially all mechanistically simple chemical reactions has been made available by recent work in computational chemistry, complementing chemical expertise, and allowing the network itself to be inferred. Because only raw formulas are accessible through measurements, the general inference framework is that of a hidden Markov model (HMM), for which a large panel of techniques have been developed, including expectation-maximization (EM) and
variational methods.
​​
Outcome. Using the fitted model will make it possible to numerically investigate, at a very low computational cost, a large variety of experimental set-ups, and hopefully give access to time scales beyond the duration of experiments, providing insights about plausible chemical evolution processes of organic matter found on asteroids and planets in their early days after their formation.
​​
Candidate. We are looking for a highly motivated mathematician or theoretical physicist with a background in statistical inference and/or statistical physics, and strong interest in applications and interactions with scientists from very different backgrounds. Some proficiency in algorithmic programming in Python is required.
​​
Situation. The project will be hosted at IECL (Institut Élie Cartan de Lorraine), which is the mathematics laboratory of Université de Lorraine, Nancy, France. The monthly net salary is ca. 2000€. Starting in Fall 2025 or beginning 2026, depending on funding and at the convenience of the selected candidate. The candidate is expected to interact strongly with experimental partner teams in chemistry and astrochemistry in Marseille (PIIM) and Poitiers (IC2MP), and with mixed theoretical/experimental close collaborators in Paris (ESPCI), all part of CNRS-funded program PEPR Origins.
​
Contact. Send a CV and cover letter to jeremie.unterberger@univ-lorraine.fr and ulysse.herbach@univ-lorraine.fr. Do not hesitate to reach us for further information.
​References:
Nghe P., Unterberger J. (2022). Stoechiometric and dynamical autocatalysis for diluted chemical reaction networks, J. Math. Biol. 85.
Nandan P., Nghe P., Stuyver T., Unterberger J. A parametrization of kinetics of organic chemistry reaction mechanisms, work in progress.
Parikh N., Boyd S. (2014). Proximal algorithms, Foundations and Trends in Optimization 1.
Robinson W. E., Daines E., van Duppen P., de Jong T., Huck W. T. S. (2022). Environmental conditions drive self-organization of reaction pathways in a prebiotic reaction network, Nature Chemistry 14.
Unterberger J. Optimal multi-time-scale estimates for diluted autocatalytic chemical networks (preprint).